
ReLambda Successfully
Implemented
'Smart AutoScaling' for
A Growing EdTech Company
Resolved the immediate challenges of degraded performance
during usage spikes as well as established a more robust and
scalable infrastructure.
during usage spikes as well as established a more robust and
scalable infrastructure.
Our client, a prominent EdTech company and rapidly growing
online education platform, faced performance degradation issues
during sudden spikes in user activity. The standard CPU-based
auto-scaling mechanism they previously relied on was insufficient
to maintain the required response time, causing latency and user
experience problems.
online education platform, faced performance degradation issues
during sudden spikes in user activity. The standard CPU-based
auto-scaling mechanism they previously relied on was insufficient
to maintain the required response time, causing latency and user
experience problems.
By focusing on observability, intelligent triggers, and advanced
scaling technologies, ReLambda successfully optimized the
client’s system, delivering a vastly improved user experience and
ensuring greater resilience in handling future growth and
fluctuations in user demand.
scaling technologies, ReLambda successfully optimized the
client’s system, delivering a vastly improved user experience and
ensuring greater resilience in handling future growth and
fluctuations in user demand.
CHALLENGES FACED BY THE CLIENT
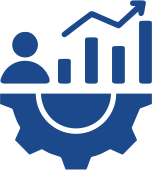
Degraded Performance:
Sudden surges in user activity led to degraded
system performance, impacting response times and
user experience.
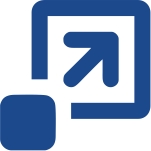
Inadequate Auto-Scaling:
The existing CPU-based auto-scaling approach
failed to effectively manage the load fluctuations,
resulting in latency issues during peak usage.
ABOUT CLIENT:
An Edtech company that offers
live online classes with gamified
peer learning activities. It
operates globally, connecting
students and teachers from
diverse backgrounds. The
company values collaboration,
openness, diversity,
adaptability, and
responsiveness to change. Over
12 million students and 100,000
teachers in 8 countries enjoy its
open learning platform, which
continues to grow.
live online classes with gamified
peer learning activities. It
operates globally, connecting
students and teachers from
diverse backgrounds. The
company values collaboration,
openness, diversity,
adaptability, and
responsiveness to change. Over
12 million students and 100,000
teachers in 8 countries enjoy its
open learning platform, which
continues to grow.
Industry
Edtech
Years in Business
10+ Years
Company Size
300+ Employees
Geographical Presence
MENA
SOLUTION BY ReLambda:
ReLambda, a cloud services provider specializing in optimized scaling solutions, addressed the challenges
for EdTech client by implementing a ‘Smart AutoScaling’ strategy:
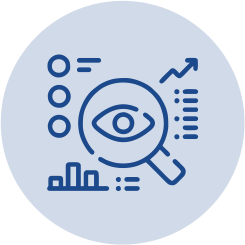
Observability Enhancement:
ReLambda integrated strong
observability functionalities to
enhance understanding of
user behavior, system
metrics, and load changes.
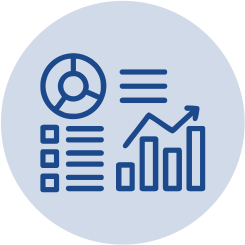
Compound Metric
Auto-Scaling Trigger:
ReLambda implemented
composite metrics blending
CPU, user activity,
resource usage, and
system health to drive
auto-scaling.
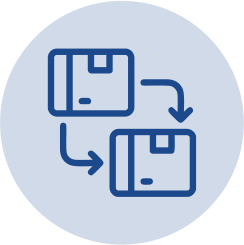
Replacement of Cluster-
autoscaler with
Karpenter:
ReLambda implemented
Karpenter, an upgraded scaling
solution for Kubernetes,
enhancing resource allocation
and scaling precision.
IMPACT:
The implementation of ReLambda’s ‘Smart AutoScaling’ solution resulted in significant improvements:
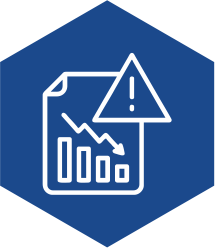
75% Reduction in Latency
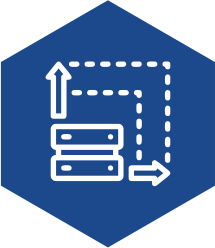
Enhanced Scalability
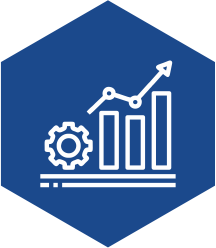
Improved Reliability
TECHNOLOGY USED:
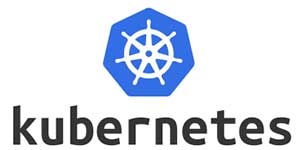
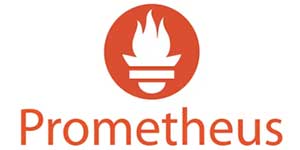
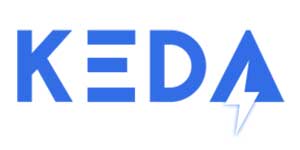
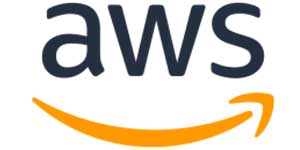
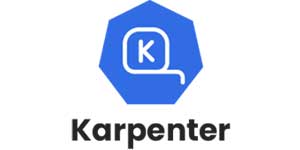
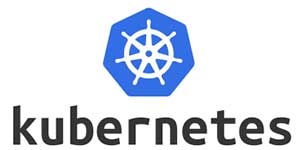
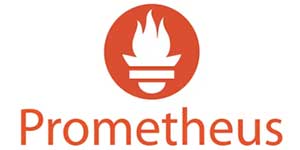
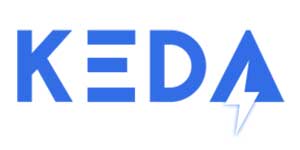
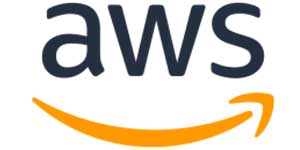
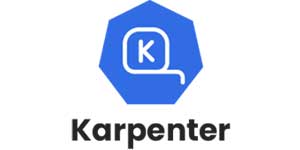